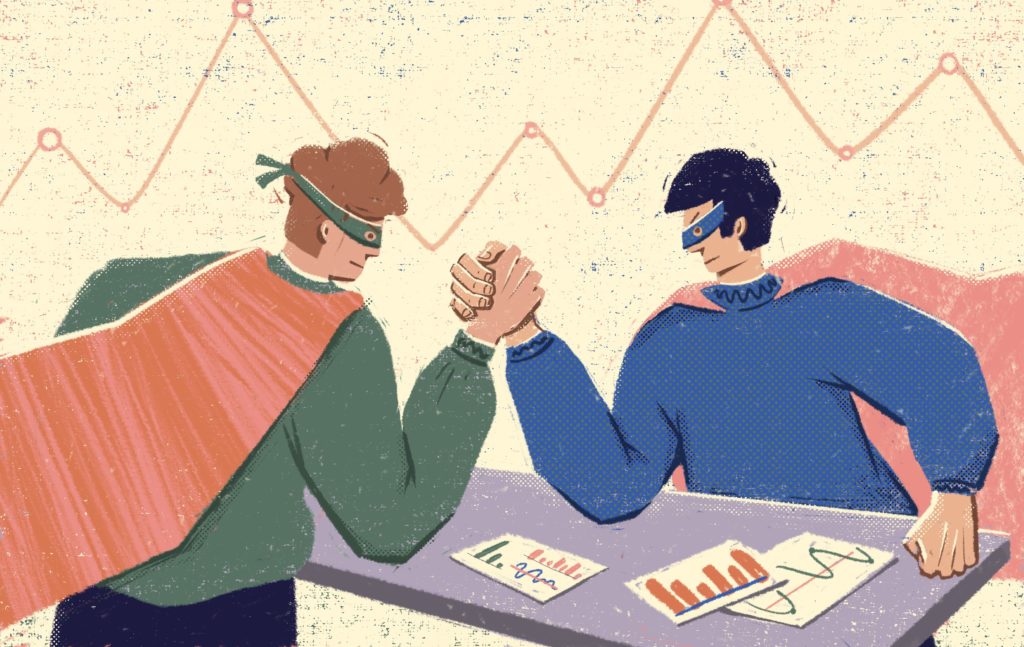
Zero-Stack vs. a Full-Stack Data Scientist
We live in an era of uncertainty. It’s uncertain what will happen to the economy in the aftermath of COVID-19 (fast/slow recovery and where and which industry sectors will be more affected). It’s uncertain how technical people will be working from now on (physical vs. remote sites), and of course, it’s still uncertain what exactly a Data Scientist (DS) is and should be (and shouldn’t be) doing in the industry.
Data Science? Not my favorite nomenclature…
The well-known academic researcher Peter Flach (10 years Editor-in-Chief of the Machine Learning journal) has recently published an article where he states that Data Science is not a very good nomenclature for the field.
The main reason for this, he feels, is that “Data Science” is prone to misguided interpretations, assuming that Physicians, Biochemistricians, or Civil Engineers are Data Scientists if they work intensively with data (aka being data-driven). Thus, Prof. Flach prefers the term “Science of Data”, defining it as follows:
“ (…)subject that studies data in all its manifestations, together with methods and algorithms to manipulate, analyze, visualize and enrich data. It is methodologically close to computer science and statistics, combining theoretical, algorithmic and empirical work (…)”.
Nevertheless, there is a trend in the industry that is pushing “full-stack data scientists”. The number of articles out there that support this trend is numerous – here is just one example.
“Full Stack data scientist” is just another facet of the AI hype
According to this trend, these mythological individuals should be capable of:
- Understanding business problems,
- Performing root cause analyses and deriving hypotheses (as a generic Big3 strategy consultant would do),
- Prepare all the data that they will need + the data pipelines needed to put something in production in the cloud,
- Create model(s), validate model(s), deploy model(s), monitor the model(s) in production from the perspective of:
- DevOps (is the service working/scaling properly?),
- A business (is it delivering the expected target KPIs?)
- A scientist (is it generalizing well? is there any concept drift?)
- An engineer (has the data input the expected format?)
And, of course, be able to present the expected/obtained results to a heterogeneous audience of stakeholders in a concise and understandable way.
Finally — and this one is the most important skill — a data scientist must be able to fly! 🙂
Naturally, this generalist DS view is not shared by all as these people tend to be very rare – and, if they do exist, they should not be staff/team member level data scientists but leaders instead.
This new full-stack DS hype raises the expectations of what AI Experts/Data Scientists (terms which I use interchangeably as a convenience, but are not quite the same thing) can and should deliver to unrealistic levels. In short, “full-stack data scientists” are just another facet of the AI hype. And, as other sectors of our society have been showing to us, history tends to repeat itself — in this case, the risk of facing yet another AI Winter, soon.
Mayday, mayday…we need Data Scientists to do Data Science!
“If I had an hour to solve a problem, I’d spend 55 minutes thinking about the problem and 5 minutes thinking about solutions.”
Albert Einstein
Data Scientists must be good at doing Data Science. Data Science problems are already difficult to solve…and imagine you a) are not a specialist and b) still need to care for all the stuff around the production of data science all by yourself. Sounds tough…doesn’t it?
This lack of understanding of what is required for a Data Scientist to be successful poses a challenge for employers looking for these experts. It’s equally challenging for the Data Scientists themselves who are looking for a position in which they can do what they do best, with realistic expectations. This is where the sorrow narrative of a full-stack data scientist starts being compelling to the masses.